Most industry insiders realize that drug development uses ML (machine learning) and AI (artificial intelligence). Researchers have utilized computational methods to locate hits, simulate drug-protein interactions, and estimate response rates for over a decade.
AI and machine learning may make drug discovery faster, cheaper, and more effective. Despite skepticism, most experts expect these techniques to become increasingly significant.
Scientists have obstacles and possibilities, especially when the processes are automated. Early-career researchers must understand AI's capabilities and how to obtain the skills needed for tomorrow's employment environment.
The AI Potential
Recent technological improvements have made capturing and storing massive amounts of digital patient data easier. AI is used in CDMO cell line development to create more efficient DNA or RNA sequences with higher affinity. This has created vast amounts of genetic data, health records, medical imaging, and other patient data that AI systems may use to produce medications faster and with a higher likelihood of success.
Offers Value
Biotech corporations need blockbuster drugs to break even. Average capitalized pre-launch R&D expenses ranged from $161 million to $4.54.
AI savings may be valuable. Investors will need to establish real-world use cases for AI-enabled drug discovery due to the substantial risks of producing physiologically plausible medicines and the short history of the technological platforms.
Three AI drug discovery tips
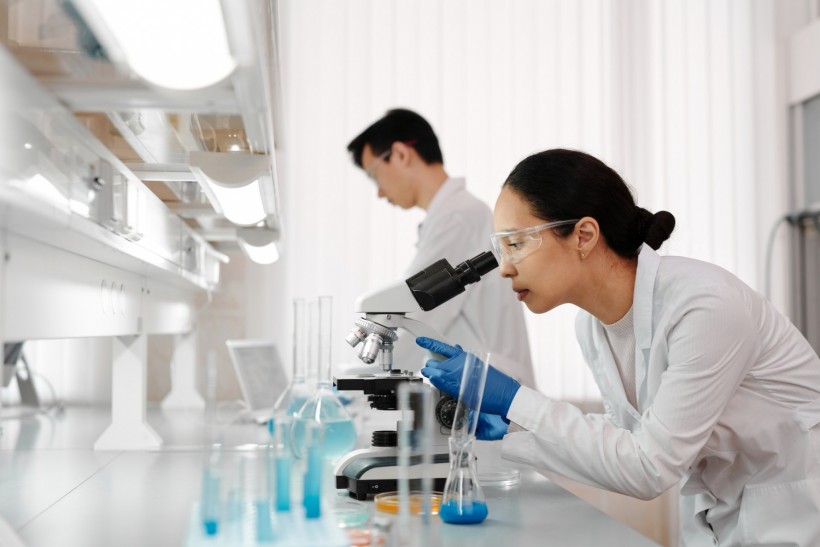
Drug discovery AI requires fair expectations, clear data, and teamwork.
Assumptions
AI can enhance a drug discovery effort. It's one tool in a box for now. Clarifying AI use is important yet difficult.
Unlike biologics, tiny molecules are simpler and have decades of data, making them ideal for AI.
AI has enormous promise, but good things take time. Pharmaceutical Technology asked its readers to estimate how long AI will peak in drug research, and the most common response was more than nine years.
Information
Curating and collecting heterogeneous, noisy, and scarce experimental data is the biggest obstacle to constructing accurate and useful AI models.
This 2021 Expert Opinion on Drug Discovery remark emphasizes the need for clean data. It applies beyond ADEMT and activity prediction models. AI needs lots of factual data.
Accuracy in data
Due to insufficient public data, companies must use their experimental data and domain expertise. Due to rising data quantities, old software, incompatible lab systems, or dispersed research teams, many firms need help to acquire, federate, mine, and prepare their data.
Collaboration
AI-using companies require a complete picture of their data. This requires a research infrastructure that connects computational and experimental teams and shares data across fields and places. Repeatable AI findings need careful process and methodological standardization. Security and secrecy are important because industry leaders work to help AI realize its full potential.
Final Thoughts
AI methods used in drug development bring the drug development process and the usage of diverse models closer to mathematicians than AI techniques used in drug development do to medicinal chemists. AI is already playing a huge role in the pharma industry, helping with drug discovery. The future of drug discovery seems bright with such an advanced resource at hand!
* This is a contributed article and this content does not necessarily represent the views of sciencetimes.com