Deep learning models have been trained to perform specific tasks—and one has been developed to achieve superhuman performance in the popular driving video game Gran Turismo Sport.
Similar to models that have learned to play chess, shogi, or go, this deep learning model was used to assess the capabilities of a deep reinforcement learning-based approach. Researchers from the University of Zurich, together with SONY AI Zurich, have developed the model that learned to play Gran Turismo Sport - the 2017 racing video game developed by Polyphony Digital and Published by the Sony Interactive Entertainment company for the PlayStation 4 platform.
RELATED: Retired Chess Grandmaster, AlphaZero AI Reinvent Chess
Their findings are pre-published on the open-access research repository arXiv, featuring the potential of deep learning techniques in driving inside simulated environments.
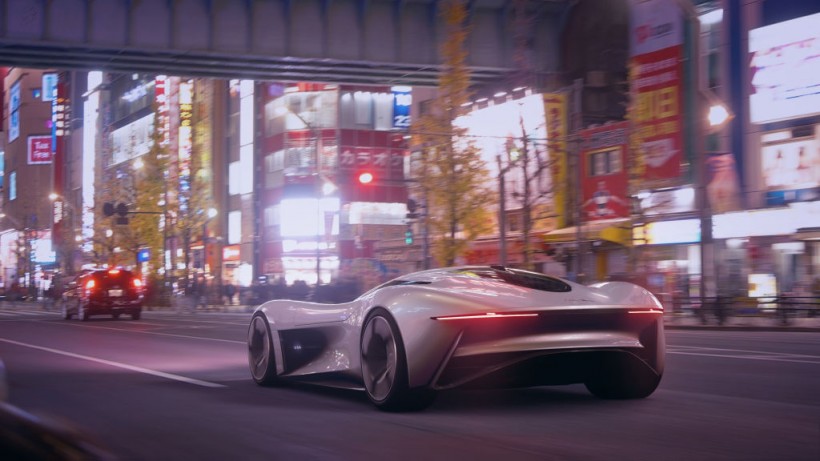
TOKYO, JAPAN - OCTOBER 25: (EDITOR'S NOTE: This image was computer generate in-game) The Jaguar Vision is seen in-game as part of the Jaguar Vision Gran Turismo Announcement prior to Round 5 of the Gran Turismo World Tour 2019 held at Future Expo Mega Web on October 25, 2019, in Tokyo, Japan.
An Artificial Neural Network-Based Video Game Driver
"Autonomous driving at high speed is a challenging task that requires generating fast and precise actions even when the vehicle is approaching its physical limits," said Yunlong Song, a Ph.D. student at the Robotics and Perception Group at the University of Zurich.
In an interview with online tech portal TechXplore, Song added that in autonomous racing, where the goal is to complete a track as fast as possible, features challenges of maneuvering a car close to its physical limits. He explained that they chose the game Gran Turismo Sport for their study because of its quality physics simulations in car models and track environments.
The study aims to develop an artificial neural network (ANN) based controller that can drive a race car on its own, even without prior knowledge of the dynamics of the vehicle in a simulated environment. As a player of Gran Turismo Sport, its performance will be measured in its runtime—minimizing the time needed to drive through a race track.
To work their model towards the goal, researchers modeled a reward function that equated the racing requirement into a "minimum-time problem." They then outlined a neural network policy mapping input observations to gameplay commands. Next, the ANN parameters were trained through reinforcement learning, i.e., a "reward" is given to the model in exchange for a "good" performance.
The experimental setup to train the ANN-based model included four PlayStation 4 gaming consoles and one desktop computer. After 73 hours of training, the deep learning Gran Turismo model was already better than most players.
Surpassing Non-Player Character and Actual Human Players
The artificial neural network was developed without receiving explicit instructions from human developers, such as intervention or path planning. Song explained that their model generates trajectories almost similar to those taken by the best human players. The study also revealed that their model had outperformed the "best known human lap times in all three of our reference settings, including two different cars on two different tracks."
RELATED: AI May Also Need Sleep Just Like Humans After Long Hours of Unsupervised Learning: Here's Why
To gain an insight into the qualitative performance of their model, researchers interviewed an expert human player of Gran Turismo Sport - one who has posted records and has played for national and international video game contests for the video game. The professional player, who remained anonymous, was asked to play with their ANN model and assess its performance.
After a few trials, the player said: "The policy drives very aggressively, but I think this is only possible through its precise actions." He added that technically, he can follow the same trajectory as the model, but noted that "in 999 out of 1000 cases," his attempts will end up in wall contact, which will severely impede the attempts to set a good time for that particular attempt.
Check out more news and information on Tech & Innovation on Science Times.