Russian chess grandmaster Vladimir Kramnik is working with DeepMind's chess program, AlphaZero, to analyze new variants in an attempt to reinvent the popular strategy board game.
Vladimir Kramnik, Classical World Chess Champion from 2000 to 2006, has proposed nine new chess variants last Wednesday, September 9. He has worked together with the artificial intelligence (AI) laboratory DeepMind, a subsidiary of Google's parent company Alphabet Inc, to evaluate his proposals with the help of the AlphaZero AI.
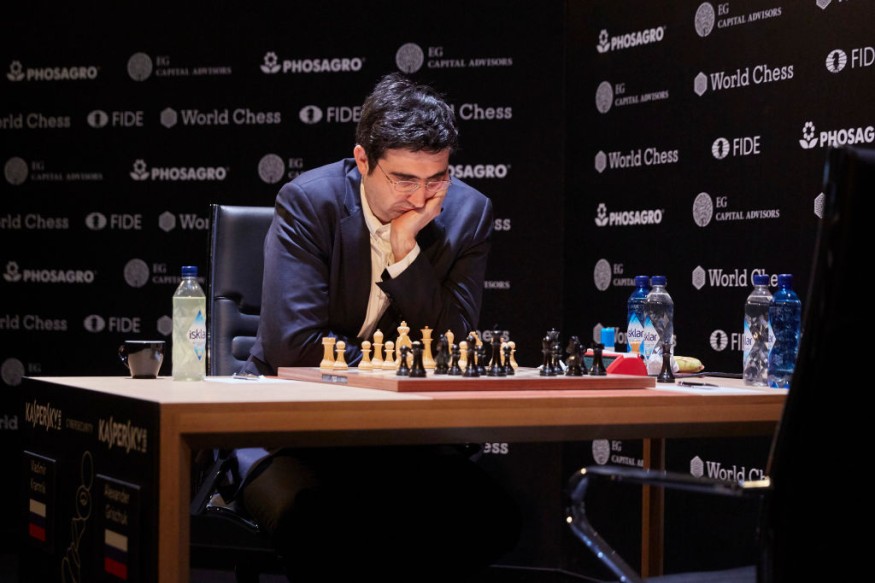
Exploring Alternative Rule Sets in Chess
In a report submitted to Cornell University, Nenad Tomašev, Ulrich Paquet, and Demis Hassabis from DeepMind worked with grandmaster Vladimir Kramnik to assess game balance in the new variations with help from AlphaZero.
It defines AlphaZero as "a reinforcement learning system that can learn near-optimal strategies for any rules set from scratch without any human supervision, and provides an in silico alternative for game balance assessment."
RELATED: Google's AlphaGo Will Have A Man vs Machine Rematch in Ancient Game of Go
The reinvention of chess involved introducing nine different alterations to existing rules of modern chess. These alterations are intended to keep the new game close to the original but allowing the generation of novel strategies and gameplay patterns. Proponents of the study aimed to preserve the appeal of the original while trying to "uncover dynamic variants" in the opening, mid-game, and endgame stages. The alterations, as a rule, do not tweak the board itself, the number of pieces, or their starting arrangements.
Some of the alterations introduced in the study include a ban of the castling move throughout the game, setting a victory condition for the side that forces the stalemate, and allowing capture of one's own pieces.
AlphaZero, which draws from a deep neural network in analyzing the move possibilities at any given chessboard condition, uses the Monte Carlo tree search (MCTS) to assess board positions. The AI program was trained by DeepMind scientists to adopt each of the rule alterations, with their computational models accommodating 1 million training steps.
In a bid to explore new frontiers in chess, our researchers worked with Vladimir Kramnik to use AlphaZero to test nine new variants of chess. The result? A more creative and collaborative relationship between chess players and machines. https://t.co/5ZQxjBrVIr via @Wired https://t.co/V54MTsQrNF — DeepMind (@DeepMind) September 10, 2020
Small Changes Lead to Large Variations in Gameplay
After simulating human play adapted to the revised rules, finding variants that appear dynamic and interesting. Furthermore, the importance of rule design on the overall dynamics of the game.
In the report's introduction, proponents explain that "Rule design is a critical part of game development, and small alterations of game rules can have a large effect on the overall playability and game dynamics."
The Russian chess GM has also provided his assessment for each of the proposed variants, with data provided by the AlphaZero AI. For example, a chess variant that does not allow castling all throughout was "a potentially exciting variant" for the grandmaster, mainly because it increases the risk for both players' kings. Removing the rook-supported strategic move allows for added complexity in the opening stages of the game.
RELATED: Complete Set of Viking Chess Unearthed in Lincolnshire, Will Be up for Sale Next Week
Another scenario, which allows a player to capture his own pieces, opens new avenues in gameplays that involve sacrificing pieces, with Kramnik noting the "aesthetic appeal" involved with giving up pieces in exchange for a strategic advantage. As the variant can feature in any stage of the game, it can appear in a large number of games, even occurring multiple times in one play.
Aside from Vladimir Kramnik, the team also tapped other chess grandmasters such as the Danish GM Peter Heine Nielsen and English GM Matthew Sadler who provided feedback on the study.
Check out more news and information on Artificial Intelligence on Science Times.
© 2025 ScienceTimes.com All rights reserved. Do not reproduce without permission. The window to the world of Science Times.