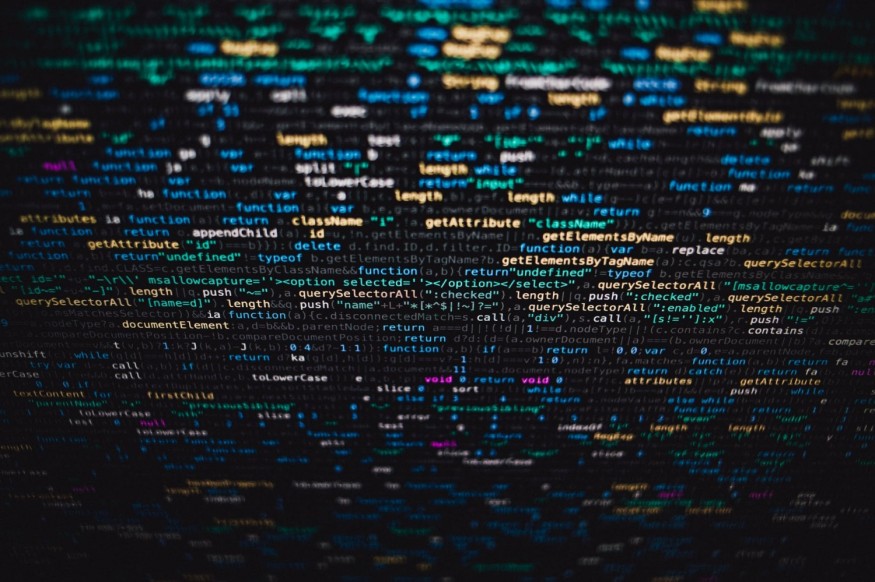
With the huge impact that artificial technology is making in healthcare in recent years, it's a big possibility that deep learning could be used to diagnose patients in the near future.
Published in The Lancet Digital Health, the review looks at how deep learning models compared to healthcare professionals in terms of medical imaging diagnosis. With over 30,000 studies identified from January 2012 to June 2019, of which only 14 were explored in-depth, the study looked at how these AI models differed from humans in sensitivity and specificity of identifying diseases through medical imaging. In this case, sensitivity refers to the probability of correctly identifying those with a certain condition, while specificity refers to the probability of correctly identifying those without the condition.
It was found that deep learning models have almost equivalent sensitivity and specificity as healthcare professionals in medical imaging diagnostic accuracy. AI had a pooled sensitivity of 87.0% compared to humans' 86.4%. In addition, deep learning models' pooled specificity had a value of 92.5%, in contrast to the healthcare professionals with a value of 90.5%.
What does this mean for healthcare professionals and the field of medicine? What would be the role of these practitioners?
For starters, it makes their job a lot easier and faster. With access to deep learning models that can automate medical diagnosis, healthcare professionals will now be able to process large volumes of data at much greater speed. With these tools at their arsenal, they can make an accurate diagnosis at a fraction of the time it normally would, possibly even in real time.
This AI also provides safeguards in medical diagnostics. Not only can this technology provide confirmatory data to initial readings done by these professionals, but it can also catch things that might be missed due to human error, providing a second barrier of defense and a safety net for these diagnostics.
This technology can also bring down the costs of healthcare, especially in developing countries. "By transferring the burden of diagnosis from people to software, global access to primary care will increase", writes David Kelnar in the MMC Ventures Report on The State of AI. Normally, medical professionals would require years of time-consuming and expensive training to become accurate with these types of diagnostics. With access to deep-learning AI, these developing countries can now receive primary care at a lower cost.
However, it's also important to note the lack of high-quality data obtained from the studies, which shows a need for better reporting design. Prof. David Curtis gives insight into this: "I think the most striking aspect is that out of over 20,000 studies of applications using AI for medical imaging published in scientific journals, only 14 were good enough to use. That's fewer than 1 in a thousand. Almost all published studies of AI for medical imaging did not use proper methods and can be safely ignored."
While deep learning AI is quite a long way off from fully replacing human healthcare professionals, this innovation provides a tool to make primary healthcare cheaper, faster, and safer when paired with trained specialists.