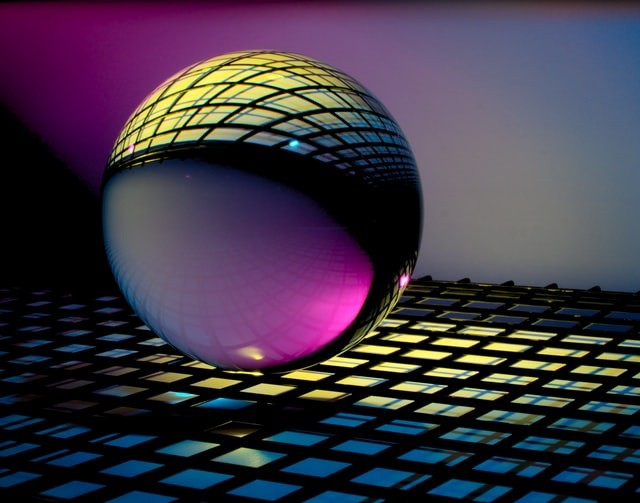
Artificial intelligence (AI) is not new to us. It has made its integrations into human life - in our phones, smart televisions, cars, healthcare, security, and almost everything. However, it is still early to say that artificial intelligence has taken over human life. We still have a long way to go for AI-based models to analyze and process things better than a human does. To make this possible, the majority of AI companies need data annotation services to speed up the deployment of these systems.
In order for AI-based models to operate at top performance, the algorithms that run them need continuous testing and learning. These algorithms continuously need relevant and consistent high-quality data. With the continuous demand for accurate data, AI companies resort to image annotation outsourcing to ensure that they get consistent, high-accuracy data. Quality training data means both the accuracy and consistency of annotated and labeled data is made possible. High-quality training data ensures more accurate algorithms and also helps mitigate the potential bias in many AI projects. Unfortunately, this level of quality at a massive scale is where the bottleneck is.
The entire point of integrating AI into human life is for it to assist humans - to analyze data, make calculated predictions, and help them build a brighter future. From acquiring information to making business decisions, AI can be used to shape the course of human life. Some machine-heavy industries such as energy, mining, agriculture, manufacturing and production will also embrace machine learning (ML) powered robotics out of pure necessity as more and more industries have adopted AI into their operations in order to innovate and disrupt their industry. As the world and society keeps on changing, AI can be used to detect societal behavior and predict the effects of policies. Additionally, to help mitigate the spread of the pandemic, AI could help detect and manage the spread in crowded areas such as schools, shopping malls, restaurants, airports, and hospitals.
As changes keep happening around the world, there will be an abundance of unprocessed data and challenges for AI to solve. The largest roadblock AI companies are facing is the volume and quality of the data required to train their AI models. According to a new report "Artificial Intelligence and Machine Learning Projects Obstructed by Data Issues" conducted by Dimensional Research, "96% of companies surveyed stated they have run into training-related problems with data quality, labeling required to train the AI, and building model confidence."
Furthermore, AI companies quickly burn through their budgets and delay their production by attempting to label and annotate training data on their own. When internal teams annotate data, there is a high chance that they will add some bias stemming from their expectations about how their model should perform. This also stalls their production because internal teams are usually composed of data scientists and engineers that are under immense pressure to deliver quality data at the required scale.
According to the said report, "71% of teams ultimately outsource ML project activities and that teams that outsource data labeling and annotation get their AI projects into production faster." While outsourcing is a great way to deploy AI projects right away, there are chances that you
might work with an outsourcing provider that is not fit to deliver high-quality data at scale. Feeding your model with bad and inconsistent data will severely affect the performance of AI.
It's important to account the following in finding the right outsourcing partner that fits your requirements:
● Quality and Expertise. In order to deliver high-quality data, the annotators you should be working with should be experienced in working with AI companies. The annotation and quality-assurance processes of data annotation involves multiple layers testing and auditing. Strict QA implementation is a vital component of your AI initiative to make sure everything is accounted for. It's also important that annotators have clearly defined guidelines to correctly annotate and label data, but not know what the data will be used for, which can influence bias on your data. This is why AI companies should prioritize partnering with a long-term outsourcing partner to get the most accurate and consistent data. In a whitepaper "Be (More) Wrong Faster - Dumbing Down Artificial Intelligence with Bad Data, it states that "if data quality is not continuously and automatically maintained, the data that was of sufficient quality at a given time for a given purpose will very quickly decay."
● Scalability. Training data must be voluminous and accurately labeled and annotated with the same level of accuracy and precision. Building AI models need continuous learning and refining of data. Varying qualities of training data might affect the performance of your AI model. Timeline. The entire reason for outsourcing data annotation is to implement your AI initiatives into production faster. Make sure that your outsourcing provider can deliver the highest quality of data at your deadline.
● Outsourcing method. There are several service providers in the data labelling industry. Some have created their own data labelling and annotation tools, while others deploy humans to annotate data. The crucial factor is how your outsourcing partner handles and annotates your data. If you will be working with inexperienced annotation teams or crowdsourced teams where quality assurance is not strictly implemented, then it might affect the overall quality of the service you invested in. The right outsourcing partner should have worked with several AI companies before to know exactly what they're doing.
Whether AI companies take the data annotation operations in-house, crowdsource, or outsource, the effectiveness of their AI strategies is heavily hinged on the preparation and the quality of data delivered for your AI systems to take off.
© 2025 ScienceTimes.com All rights reserved. Do not reproduce without permission. The window to the world of Science Times.