A new study published in the Nature journal investigates photonic processors' potential for applications of artificial intelligence or AI.
According to Tech Xplore, such photonic processors allow computers to complete complex computations at incredibly fast speeds.
For the first time, the results exhibit that these tools can process information fast and in parallel, something that the present day's electric chips are not capable of doing.
According to electrical and computer engineering assistant professor Nathan Youngblood, from the University of the Pittsburgh Swanson School of Engineering, 'Neural networks are learning by taking large sets of data and identifying "patterns through a series of algorithms."
Youngblood, the study's co-lead author, added that this newly introduced processor would enable it to "run multiple calculations" simultaneously by using different optical wavelengths for every computation.
The study investigators wanted to solve the problem: incorporation, specifically, how to do calculations using light in an efficient and scalable manner.
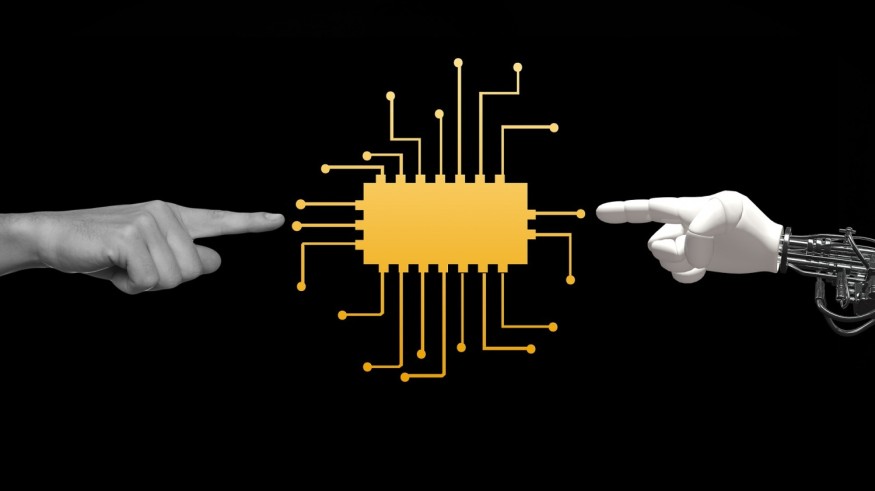
Fast and Efficient Processing
The study investigators' fast and efficient processing is perfect for applications like self-driving vehicles, which need to process the data sensed from several inputs as fast as possible.
Furthermore, photonic processors can support cloud computing applications, too, and medical imaging, among others.
University of Munster's senior co-author Wolfram Pernice said light-based processors for fast-moving tasks in the field of AI allow complex computational tasks to be "processed at high speeds and throughputs."
He added that this is much speedier than conventional chips, which depend on a transfer through electronic data like graphic cards or specialized hardware like Tensor Processing Unit or TPUs.
The study was conducted by an international research team, including Pitt; the University of Munster; England-based Universities of Oxford and Exeter; Lausanne, Switzerland-based, Ecole Polytechnique Federale; and Zurich-based IBM Research Laboratory.
Phase-Change Materials Combined
The study authors combined phase-changed materials, the storage materials, for instance, on DVDs, and photonic structures for data storage in a "nonvolatile manner" minus the continuous energy supply requirement.
The research is a pioneer, too, in combining the optical memory cells with a "chip-based frequency comb" as a source of light, which enabled them to compute on 16 different wavelengths at the same time.
In this project, the authors applied the technology to develop a convolutional neural network that would identify handwritten numbers.
They discovered that the approach granted "never-before-seen" rates of data, not to mention calculating densities.
Graduate student Johannes Feldmann, from the University of Munster said, "the convolutional operation between input data and one or more filters," which can be an underscoring of edges in an image, for instance, can be transmitted very well to their matrix architecture.
Feldmann, also the study's lead author, added, "Exploiting light for signal transference" allow the processor to conduct parallel data processing by means of wavelength multiplexing, which results in a higher computing density to a lot of matrix multiplications "being carried out in just" a single timestep.
Opposite the traditional electronics, which typically work in the low GHz range, optical variation speeds can be attained with speeds of a maximum of 50 to 100 GHz range.
READ NEXT : Kepler Space Telescope Reveals About 300 Million Habitable Planets Possibly Exist in the Milky Way
Check out more news and information on Artificial Intelligence on Science Times.
© 2025 ScienceTimes.com All rights reserved. Do not reproduce without permission. The window to the world of Science Times.