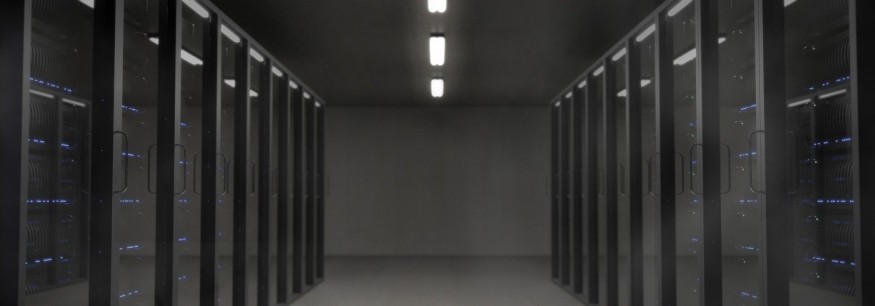
The progressive development of technology contributes to the expanding global data domain. Perhaps, the size of data created every year is expanding faster than before.
Moreover, data growth is also the birth of numerous data handling procedures, including data science and data mining services. These terms may sound similar because they both deal with data, but they are entirely different things.
The Difference Between Data Mining and Data Science
Data Mining refers to extracting essential functioning data from a more extensive set of raw data. It is also known as data discovery. It is a procedure and methodology that involves data analysis, where the focus is on identifying valuable information in a database, and using it to reveal hidden trends.
Data Science, on the other hand, refers to the field of study that unites programming skills, statistics, domain expertise, and mathematics knowledge to pull out relevant understanding from many unstructured to structured data. It is often referred to as data-driven science. It is an area or broad domain that involves collecting and processing data and obtaining information.
Historical Background
The notion of Data Mining has been around for over a century, but it caught the public's attention only in the 1930s. One of the first mentions of it was when Alan Turning establish the concept of a universal machine that could carry out computations in the same way as modern-day computers.
On the other hand, Data Science was used as an alternative term for Computer Science in the 1960s, but now has an entirely different meaning. Its history started in 1962 when John W. Turkey speculated on data processing as an empirical science in modern-day electronic computing.
Primary Roles and Key Features
Data Mining is used for knowledge extraction and model prediction generation. Therefore, it is a subcategory of data science. It analyzes data sets generated to discover inconsistencies and hidden patterns and trends from structured data.
Data Mining Key Features:
Automatic forecasts of patterns based on the study of trends and behaviors.
Prediction on the grounds of probable results.
Creation of knowledge which is decision-oriented.
Emphasis on broad scientific data sets and libraries.
Groupings focused on identifying classes of information not previously recognized and visually registered.
Data Science stems from structured and unstructured database perspectives. It is used for qualitative research as a multi-disciplinary field. Perhaps, it consists of behavioral science, data visualization, language analysis, data mining, and unstructured data and statistics.
Data Science Key Features:
Its findings can be applied to almost all kinds of sectors, such as healthcare, education, and travel.
It can help industries quickly analyze their challenges and effectively address them as well.
Organizations and their products will be able to create a fair and more in-depth understanding of how customers can use their products with data science assistance.
Uses and Purposes
Data Mining focuses on finding multi-source information and turning the data into a valuable tool. Moreover, Data Mining has many purposes. It can be used for:
Pattern detection;
Predicting different results;
target group modeling;
Collection of product/service consumption information;
Moreover, Data Mining helps to explain some aspects of the client's behavior. Perhaps, this information allows businesses to adapt and provide the best possible services continually.
Meanwhile, Data Science generates data-focused solutions for businesses and influences decisions with the support of data. Perhaps, its primary purpose is to determine correlations within data. Therefore, it requires the use of various statistical methods to evaluate and derive knowledge from the data.
For instance, knowledge from data collection, pre-processing, and maneuvering should be closely monitored by a data scientist. Then he has the responsibility to make predictions out of the data details. Also, the task of a data scientist is to derive insights from the data. In that way, he can help companies make better business choices.
Both Data Mining and Data Science can be used in different industries.
Application
In data mining, companies use the trends and patterns found to formulate processes, marketing, and economic strategies to drive business growth.
Some of data mining's main industry applications are in:
Telecommunication. It takes care of many telecommunication company management operations, such as reduced calling fees, marketing/customer profiling, customer churn reduction, network infrastructure management, fraud identification, and so on.
Business and Marketing. It analyzes data used for market segmentation, competition analysis, reducing costs, improving customer satisfaction, predicting possible risks, market intelligence, increasing sales, etc.
Finance. Data Mining helps increase revenue, reduce operational costs, enhance user experience, forecast the financial markets, and reinforce security.
Banking. It helps banks identify the critical channels of transactions, understand their customers' habits, detect and prevent fraud, segment customers, analyze customer feedback, and assess risk.
E-commerce. E-commerce businesses use Data Mining to keep their customers engaged with their website and improve their experience.
Health-care and Medicine. Data Mining is used to analyze medical records and for financial purposes like client acquisition and reducing costs.
Cybersecurity and Information. It helps identify cybersecurity risks, such as malicious insider program, infected and weak devices, and malware/ransomware attacks.
Data Science, on the other hand, is scientific research that opens the way for an analysis based on a project, program, or portfolio.
Some of the data science application examples are:
Prediction of demand for the manufacturing industry. It helps manufacturers to optimize supply chains and deliver orders without under/overdoing-ordering risk.
In the logistics sector, supply chain optimization. With the help of Data Science, logistics can predict the demand for their services, speed up their work, and reduce operating costs.
In the retail sector, customer analytics. It helps retail sectors create interest in their new products and sell out old products.
Marketing & advertisement recommendation systems. It helps stores better understand their customers' behavior and bring them closer. In this way, store owners will know what they should change to keep customers interested in their products.
Analytics on revenues. Data Science algorithms can help sales representatives decide between services or products suitable to introduce to a potential client.
Now with the help of GroupBWT you know the difference between Data Mining and Data Science, you can now choose what's best to use to improve your business. There are various Data Science and Data Mining services available in the market that you can choose from. Make sure to find the most suitable for you and your business.
© 2025 ScienceTimes.com All rights reserved. Do not reproduce without permission. The window to the world of Science Times.