Computer scientists and experts in energy technology at the Case Western Research University have teamed up to leverage artificial intelligence or AI's diagnostic power to make solar-power plants more efficient.
Solar power utilizes energy coming from the sun collected by photovoltaic or PV modules to develop clean and renewable energy.
Researchers said that making more solar-energy plants more efficient will benefit the industry and, absolutely, the consumers.
According to Solar Durability and Lifetime Extension Research Center and Kyocera Professor of Ceramic, Department of Materials Science and Engineering at the Case School of Engineering director Roger French, solar is currently the "cheapest form of electricity in the world." However, the efficacy of the actual power plants is being assessed simultaneously, and that is just not "tractable, especially for a fast-growing industry."
This study that gained three-year funding or equivalent to a $750,000 grant from the United States Department of Energy, is part of a comprehensive $130-million solar-technologies effort which the DOE announced in 2020, including $7.3 million, particularly for machine-learning initiatives, as well as other artificial intelligence for solar applications.
French and Materials Science and Engineering research associate professor Laura Bruckman are co-lead authors of the study.
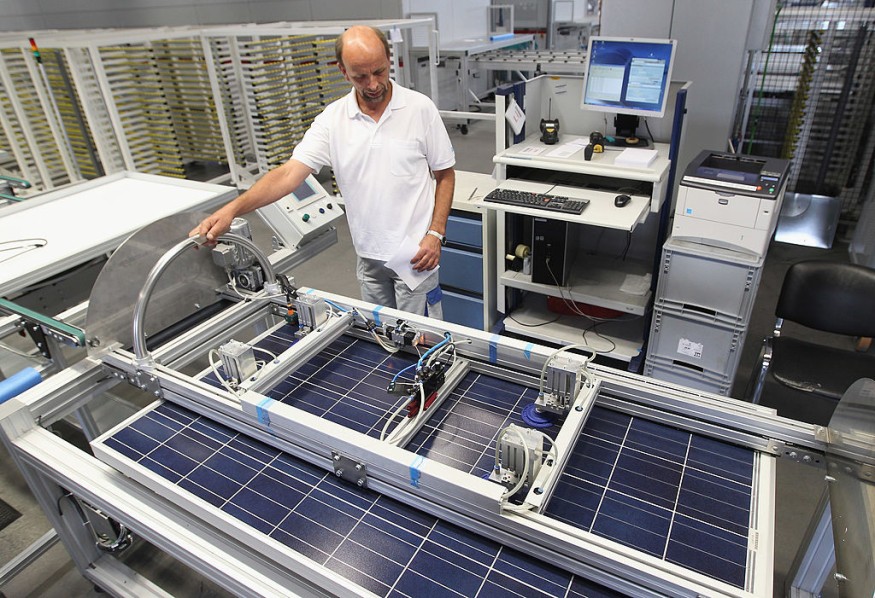
Machine Learning for More Efficient Solar Energy
Essentially, this Case Western Reserve-led study aims to use technology, specifically computers, better to assess data from a huge quantity of PV systems to help measure both their short- and long-term performance.
Those machine-learning approaches will be used to solve data-quality issues impacting the individual plants. For that to happen, the study authors say they would use a "spatiotemporal graph neural network framework."
The spatiotemporal method means determining how plants are performing differently in space and time and building a framework to improve all distinctive PV plants in that group and future systems.
Mehmet Koyuturk, Computer Sciences professor at the Andrew R. Jennings and one of the members of the team, said since there is no robot to visit all of the "photovoltaic plants to look at the info and determine patterns" of similarity between behaviors, all of the collected data are used instead, to act "as if we did."
Pre-Trained Computer Model for Solar Energy Companies
According to Bruckman, different firms have information on their own technology in their country's specific area. However, to date, "we have not had a chance to be able to gather and assess" all data from a great range of areas and companies.
Finally, the team, researchers, and Yinghui Wu, one of the team members, a Department of Computer and Data Sciences assistant professor, said the study would help the solar industry and eventually energy users, but researchers of AI, as well.
Each time a new system for understanding fresh data from specific domains is built, Wu, who's also a co-investigator on a National Science Foundation-funded research, explained, it helps understand further "our own science."
French explained, his group would work on collecting and evaluating data this year, then begin offering solar-energy firms and individual power plants a "pre-trained computer model" to analyze how to enhance their own system.'
Check out more news and information on Solar Energy on Science Times.
© 2025 ScienceTimes.com All rights reserved. Do not reproduce without permission. The window to the world of Science Times.