Breakthrough research from Google demonstrates how artificial intelligence could design computer microchips that, at the very least, perform as well as those designed by microelectronics experts - promising to be faster and more efficient in the future.
Floorplanning in VLSI systems, or the process of designing the physical layout of a microchip, including the positioning and connections between the electronic components required, is a critical step in optimizing the possible performance of a device. This requires teams of experts months of planning and design proposals and tradeoffs.
However, despite decades of study into the design and development of computer microchips, there remains no technique to uniformly execute floorplanning designs whose performances are on par with manually designed microchips.
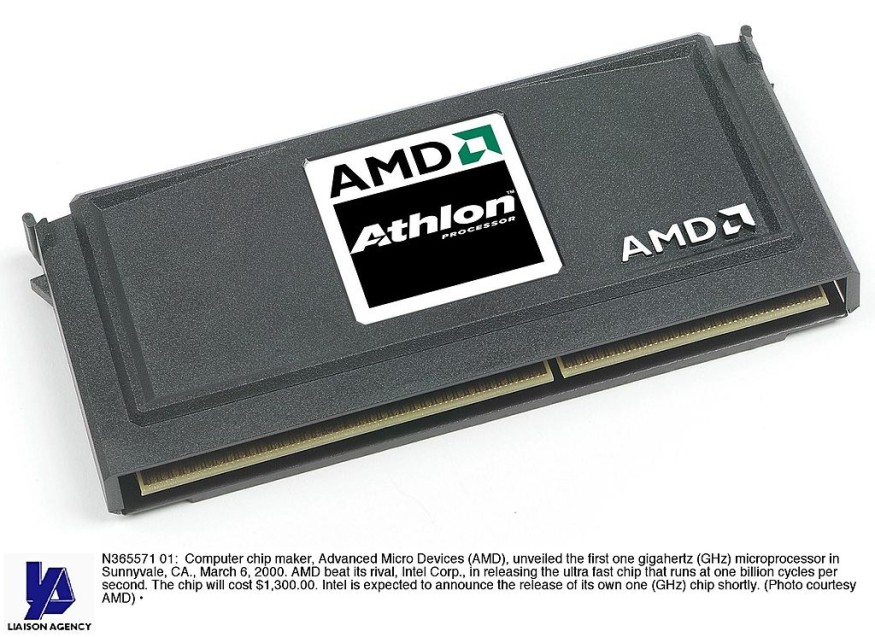
Using AI and Reinforcement Learning for Floorplanning Microchips
In April 2020, Google's Senior Software Engineer Anna Goldie and Senior Research Scientist Azalia Mirhoseini from the Brain Team published a report through the Google Blog platform. Noting how Dennard scaling, which states that while transistors tend to get smaller, their power density remains roughly constant, and Moore's Law, or the prediction that the number of transistors in microchips doubles every two years, is slowing down. This led researchers to find new ways to meet the growing need for more and more computing power.
They then pose chip placement - or the positioning and orientation of transistors and components in microchips - as a reinforcement learning (RL) problem. It is then introduced as an RL policy for an agent to be trained upon, learning the optimal chip placement upon "studying" datasets. What sets this apart is that it takes all previous attempts at floorplanning to improve over time. Additionally, researchers said that as they train the AI agent over a greater number of chip blocks, it becomes better and faster at creating optimized placements.
On June 9, researchers published the report "A graph placement methodology for fast chip design" in the latest edition of the journal Nature. The newly-published research contains updated methods on the AI floorplanning method and its application in the design of the next generation of Google accelerator chips, or tensor processing units (TPU).
Characterizing the Floorplanning Problem
Designing computer microchips is often divided into multiple blocks. Think of each block as a module designed for a specific purpose: for power distribution and regulation, computation, watchdog timer, memory, logic, and more. Each block is characterized by a netlist (a summary of the connectivity required), a graph of the components and their connections - this includes macros and logic gates - all of which are linked together by wires.
Floorplanning in microchips mainly aims to generate a design that optimizes the PPA - power, performance, and area - while keeping considerations on the overall chip size, component density, and routing restraints.
In the Google AI agent, they used the chip netlist, particularly the node types and adjacency details of the microchips, as well as the node IDs and metadata like total wire numbers, macros, and clusters. For every iteration of the reinforcement learning training, the AI agent makes adjustments following set criteria for improved metrics.
Google has begun manufacturing AI-designed microchips and believes that more powerful devices could be designed by these systems in the future.
RELATED ARTICLE : Deep Learning Model Outperforms NPC, Player Records in Gran Turismo
Check out more news and information on Artificial Intelligence in Science Times.
© 2025 ScienceTimes.com All rights reserved. Do not reproduce without permission. The window to the world of Science Times.