Machine learning algorithms have essentially become a part of humans in their daily lives. From filtering unwanted emails, improving configurations in cars, and recommending products or services. Now, scientists think that teaching an algorithm to accurately predict water temperature and streamflow could be possible.
Researchers from the University of Minnesota, University of Pittsburgh, and the US Geological Survey published a study on accurately predicting the flow and temperature of rivers.
The study, "Physics-Guided Recurrent Graph Model for Predicting Flow and Temperature in River Networks," was published in the 2021 Society for Industrial and Applied Mathematics (SIAM) International Conference on Data Mining (SDM21) proceedings. It was supported by the National Science Foundation (NSF) award.
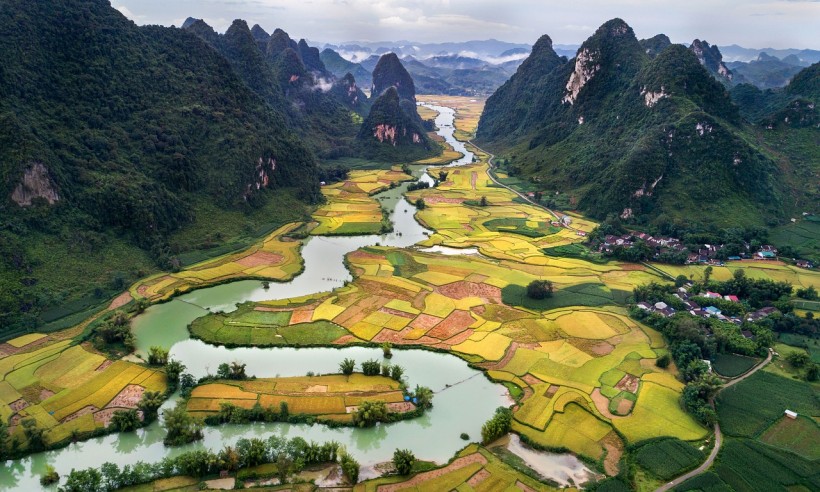
Algorithm Based on the Physical World Could Help Predict Flow and Temperature of Rivers
Combining Algorithm and Physics-Based Models
According to the journal's abstract published on the University of Minnesota's website, the research proposes combining algorithm and physics-based models to improve the prediction of the flow and temperature of river networks.
The team built a recurrent graph network model to simulate the interactions that happen in different river networks; then they transferred the data of physics-based models to guide the algorithm of the machine learning model.
The researchers propose a new loss function that balances the performance of different river segments, particularly in predicting the water temperature and streamflow in a subset of the Delaware River Basin.
They said that the proposed method improved the accuracy rate of the state-of-the-art physics-based model by 33%/14%. Meanwhile, a 24%/14% improvement was also observed in the accuracy of traditional machine learning models in predicting temperature and streamflow.
The team noted that the proposed method had shown significant performance improvements when generalized to different seasons and river segments that have various streamflow ranges.
ALSO READ: AI and Misinformation: How Artificial Intelligence Works on Both Sides
Importance of Water Temperature Predictions
The US Geological Survey released an interactive visual that highlights the model developments and the importance of water temperature predictions in the Delaware River Basin.
EurekAlert! reported that the interactive visual presentation showed the societal needs for accurate prediction of water temperature and streamflow where reservoirs provide drinking water to over 15 million people, and the water demands to maintain a downstream flow and cold water habitat for game fish species.
With an artificial neural network (ANN) that can accurately predict the flow and temperature of river networks, reservoir managers could release cold water when they anticipate that water temperatures will exceed critical thresholds and only use limited water resources when necessary.
The algorithm of the machine learning model that learned the physics-derived equations to make accurate predictions is called knowledge-guided deep learning. This model can do more with fewer data, unlike previous machine learning models.
That means even when it only has 1% temperature data for training, it can still make accurate predictions on the water temperature and streamflow.
This gives scientists the confidence to use knowledge-guided models to make predictions to even river basins with limited observations.
RELATED ARTICLE: Researchers Develop Machine Learning Algorithm to Help Further Understand Quantum Systems
Check out more news and information on Machine Learning in Science Times.