A pair of researchers from Skolkovo Institute of Science and Technology (Skoltech) has developed an efficient neural network model trained to find protein-peptide binding sites, which could significantly advance peptide-based drug discovery.
The new neural network, called BiteNetPp, uses known data from the structure of proteins to predict which parts interact with peptides, biological molecules made of short amino acid chains. Peptides have been used for drugs that make up and affect protein to protein interactions in various cells that, in turn, regulate a wide range of cellular processes.
Skoltech researchers present their findings in the Journal of Chemical Information and Modeling in the report "Protein-Peptide Binding Site Detection Using 3D Convolutional Neural Networks."
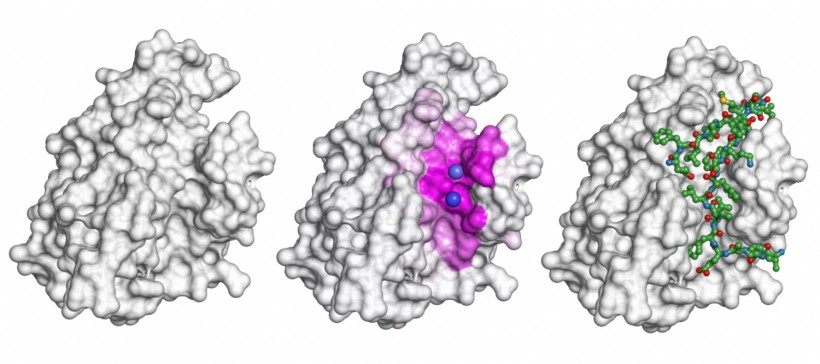
ALSO READ: Peptides Can Form Without Amino Acids
The Need To Find Potential Peptide Sites
Proteins are considered the basic machinery of the cells, moving around, connecting and interacting with each other, and affecting various bodily operations. The interactions between these structures have been of significant interest to the field of pharmaceuticals.
However, it was not as straightforward as it seems, especially since the larger therapeutic molecules, called biologics, could not penetrate deep into the cell to directly interact with proteins, as noted in a 2020 Mini Review Article on the Molecular Biology Reports. On the other hand, smaller agents usually proved incapable of achieving the same actions.
In this concern, peptides, which are involved in about 40% of cellular processes, offer a unique middle ground for specific medications intended for protein to protein interactions. Peptides, like small molecules, can enter the cell membrane and bind with the target proteins within. Also, like engineered biologics, peptides have low toxicity, high affinity, and high specificity, which creates a focused and robust action when used as a medication medium.
In designing peptide-based drugs, pharmacologists must first know the binding sites. These are specific locations in a protein that allow binding with a peptide. With more areas identified, more drug design and development opportunities are available.
There have been multiple methods for locating binding sites, most of them, experimentally. One standard method is the use of X-ray crystallography, the most favored technique according to a previous review in the Molecular Pathology journal. It reveals the 3D structure of crystallized proteins by examining how they diffract X-rays directed at them.
Meet BiteNetPp: Locator for Protein-Peptide Binding Sites
Named BiteNetPp, the new computational method uses the capabilities of 3D convolutional neural networks to locate protein-peptide binding sites. The new model is trained with a known protein structure, and BiteNetPp highlights possible binding sites. It then returns a set of potential 3D coordinates together with the probability scores for each of them.
Researchers demonstrated that the new neural network consistently outperforms other existing state-of-the-art methods by comparing BiteNetPp predictions for protein-peptide binding sites that have been identified through experimental observations.
More importantly, the Skoltech model exhibits unprecedented speed as it takes less than a second to analyze an entire protein structure, making it advisable for large-scale studies. Computational methods need to be fast enough to deliver feasible screening to be used for pharmaceutical efforts.
RELATED ARTICLE: Skoltech Leads Open-Source Project Using Neural Networks To Generate Organic Molecule Nomenclature
Check out more news and information on Neural Networks in Science Times.