Abstract:
This scientific article delves into areas of Artificial Intelligence (AI), such as Natural Language Processing (NLP) and Machine Learning (ML), and their connection to health. It starts by discussing the development of NLP and ML and how they are used to identify health-related problems. The focus then shifts to health disorders, including a modern fear called nomophobia, which refers to the fear of being without one's mobile phone. The article briefly explores the evolution of NLP and ML, their interaction, and their potential role in reshaping health diagnostics and support.
Tags: Natural Language Processing, Machine Learning, Health, Nomophobia
1. Introduction:
In the changing fields of healthcare and technology, there is an emphasis on integrating Artificial Intelligence (AI), Natural Language Processing (NLP), Deep Learning (DL), and Machine Learning (ML) when it comes to mental health. This article aims to investigate the role played by NLP, ML, and DL in recognizing, understanding, and addressing issues related to health.
In recent years, there has been a growing awareness of mental health concerns along with an increased demand for effective solutions as we gain a better understanding of the complexities surrounding these conditions. The impact of these issues on society is significant, requiring approaches to diagnosing, treating, and supporting affected individuals. Artificial Intelligence (AI), Natural Language Processing (NLP), and Machine Learning (ML) have emerged as tools in this effort. They offer the ability to analyze datasets, understand subtle language nuances, and identify signs of mental health conditions.
This article begins by explaining the importance and urgency of the topic, placing it within the context of AI's influence across industries. It then explores the principles and development of NLP and ML, emphasizing how they work together to address health challenges. Through real-life examples, this article aims to highlight the growing significance of AI-based solutions in improving health diagnosis and support.
2. NLP, ML, and DL: Foundations and Evolution
In tracing the journey of Natural Language Processing (NLP) and Machine Learning (ML), we embark on a historical odyssey that encapsulates the remarkable evolution of these fields. From their early stages in the 1950s to their present-day prominence, NLP and ML have undergone an exciting metamorphosis, culminating in the emergence of cutting-edge deep learning models. Along this journey, significant milestones and breakthroughs have shaped these technologies, elevating their capabilities. As we delve into this timeline, we will uncover moments that not only influenced NLP and ML but also revolutionized their application in understanding and supporting mental health-a testament to their ever-expanding potential and importance in our digital era.
A concise timeline illustrates how Natural Language Processing (NLP) and Machine Learning (ML) have evolved: [2, 3]
1950s - 1960s:
- NLP's early foundations are laid with attempts at machine translation and language processing.
- Rule-based systems form the basis for language processing but are lacking sophistication.
1980s - 1990s:
- Statistical approaches gain prominence in NLP, incorporating probabilistic models and corpora of text data.
- ML begins to take shape with decision trees, support vector machines, and naive Bayes classifiers.
- Early applications include information retrieval and part-of-speech tagging.
2000s - 2010s:
- Deep Learning redefines NLP and ML, mainly through recurrent neural networks (RNNs) and later transformer models.
- Models like Word2Vec and GloVe revolutionize word embeddings, enabling a better language understanding.
- NLP shifts from rule-based systems to data-driven approaches, improving machine translation, sentiment analysis, and text generation.
- ML experiences a resurgence with the widespread adoption of neural networks, achieving breakthroughs in image recognition, speech recognition, and NLP tasks.
Present (2020s):
- NLP and ML continue to advance rapidly, with models like BERT and GPT-3 achieving state-of-the-art results across various language tasks.
- In the field of mental health, NLP and ML begin to play a crucial role in sentiment analysis, language pattern recognition, and chatbot-based interventions for early detection and support.
This timeline demonstrates how NLP and ML have evolved significantly over time. They have gone from beginnings to playing a role in transforming mental health diagnostics and support today.
3. The Synergy of NLP and ML:
In the world of artificial intelligence, the close relationship between Natural Language Processing (NLP) and Machine Learning (ML) stands as a fundamental pillar. NLP, a branch of ML, acts as a bridge connecting raw language data with machine comprehension. This interdependency strengthens our ability to navigate the complex nuances of human language, empowering machines to understand, interpret, and generate textual and spoken content.
Within the changing realm of healthcare, the collaboration between Natural Language Processing (NLP) and Machine Learning (ML) has emerged as a catalyst for identifying health-related issues. These powerful AI technologies work together, supported by algorithms and extensive datasets, enabling healthcare professionals to navigate through patient data, medical records, and clinical literature with unprecedented precision and efficiency. With its linguistic prowess, NLP excels in understanding and extracting valuable insights from textual data. It can decode notes, patient records, research papers, and even social media content to uncover patterns, sentiments, and crucial health-related information. ML, on the other hand, contributes its capabilities to this partnership by allowing algorithms to learn from data and make informed decisions.
Together, NLP and ML are harnessed in several ways to identify health-related issues [1, 12]:
Sentiment Analysis: NLP is deployed to analyze text data's sentiment and emotional state. This is particularly valuable in assessing the mental and emotional well-being of individuals. For instance, sentiment analysis can detect signs of stress, anxiety, or depression in social media posts or patient narratives.
Language Pattern Recognition: ML models that have been trained on healthcare datasets have the ability to detect language patterns that are linked to particular health conditions. When there is a change in these patterns, like an increase in words or phrases, it can potentially indicate the beginning or worsening of a health problem.
Chatbots and Virtual Assistants: NLP-driven chatbots and virtual assistants engage with users in natural language conversations. They can assess users' symptoms, answer health-related questions, and identify potential health concerns. These AI-driven interactions provide immediate support and can recommend seeking professional medical advice when necessary.
Electronic Health Records (EHRs): NLP techniques are used to extract structured information from unstructured EHRs. ML algorithms then analyze this structured data to identify trends, risk factors, and potential health issues among patient populations.
Clinical Decision Support Systems: NLP and ML contribute to clinical decision support systems by processing vast amounts of medical literature, research findings, and patient data. These systems provide healthcare practitioners with evidence-based recommendations for diagnosis and treatment.
Early Detection and Intervention: NLP and ML are employed to identify early warning signs of diseases or adverse health events. For example, they can analyze patient records to predict the risk of readmission or flag irregularities in vital signs data.
Population Health Management: These technologies enable healthcare organizations to gain insights into population health trends and allocate resources effectively. They can identify prevalent health issues within specific demographics and regions, aiding in preventive healthcare strategies.
5. Mental Health Issues and Types:
In recent years, there has been an increase in the use of AI in identifying mental health issues. Figure 1 depicts the ascending trend in research for mental illness detection driven by NLP, indicating significant research value and potential for automated mental illness detection from text, along with a rise in the popularity of deep learning-based methods in recent years.
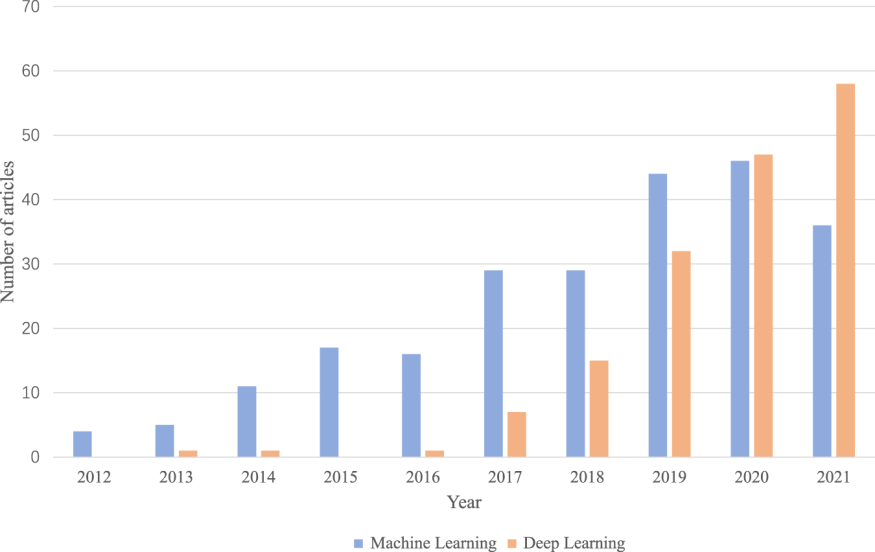
In this section, we will provide a brief overview of some examples of mental health conditions, such as depression, anxiety, and various phobias - Then, we will look at examples of symptoms and explore how AI-powered tools using ML and NLP can play a role in identifying and supporting these disorders.
5.1. Depression: Depression is a widespread and incapacitating mood disorder marked by enduring feelings of sorrow, despair, and a lack of enjoyment or interest in everyday activities.[4]
Examples:
Major Depressive Disorder (MDD): An individual suffering from Major Depressive Disorder (MDD) may display a continuous low mood, a lack of energy, alterations in appetite or sleeping patterns, and challenges in maintaining focus.
Postpartum Depression: Occurs after childbirth and is marked by feelings of extreme sadness and fatigue, impacting a mother's capability to nurture/care for her child.
Symptoms:
Common symptoms include:
Persistent sadness
Loss of interest or pleasure in activities
Changes in appetite or weight
Sleep disturbances
Fatigue and low energy
Feelings of worthlessness or guilt
Difficulty concentrating or making decisions.
Thoughts of death or suicide.
NLP Utility: NLP and ML can analyze text data, such as social media posts or electronic health records, to detect linguistic patterns associated with depression. Sentiment analysis and language pattern recognition can provide early warning signs, allowing for timely intervention and support. [4,5]
5.2. Anxiety Disorders:
Description: Anxiety disorders encompass a range of conditions characterized by excessive worry and fear [7]
Examples:
Generalized Anxiety Disorder (GAD): People with GAD experience chronic and excessive worrying about various aspects of life.
Social Anxiety Disorder: This condition involves an intense fear of social situations and scrutiny by others.
Symptoms:
Common symptoms include:
Excessive worrying
Restlessness
Muscle tension
Rapid heartbeat
Shortness of breath
Sweating
Trembling
NLP Utility: AI-driven chatbots and virtual assistants can engage with users to assess anxiety levels and provide immediate support or guidance [7, 8, 9]. NLP can analyze text data, such as chat logs or social media posts, to identify individuals expressing anxiety-related concerns [6].
5.3. Phobia:
Description: Phobias are intense and irrational fears of specific objects, situations, or activities. Examples:
Nomophobia: A modern phobia characterized by the fear of being without one's mobile phone- or, more generally, the fear of being separated or being disconnected from technology [10].
Symptoms: Anxiety or panic without a phone caused by having access to any smart device. Excessive use/ smartphone addiction and sleep disruptions/insomnia [10, 13]
NLP Utility: NLP can be used to analyze social media content, text messages, and other digital interactions to identify expressions of phobias like nomophobia [10, 11]. This information can aid in understanding the prevalence and impact of modern phobias and provide support or interventions when necessary.
5.3.1 Nomophobia case study:
In this section, we delve into the rise of nomophobia-a concern that is becoming increasingly prevalent in our digital era. We will examine how natural language processing (NLP) and machine learning (ML) technologies can be utilized to recognize these fears. Through this exploration, we shed light on approaches to comprehending and supporting individuals who struggle with phobias in the 21st century.
5.3.1.1 Introduction:
Nomophobia, a term derived from "no mobile phone phobia," is becoming a growing issue that highlights a reliance on mobile phones. A notable study by Vaishnavi Visweswaraiah et al. explored the impact of nomophobia before and after the COVID-19 pandemic, utilizing social media data from Twitter to gain insights into how expressions of dependency on phones have changed during this time period. [10].
5.3.1.2 Methodology:
The study examined tweets from February 2020, before the COVID-19 pandemic, and May 2020, during the COVID-19 era. There are two stages in this study: 1) To determine if tweets were related to nomophobia or not, a relevancy classifier was built using machine learning algorithms such as regression, naïve Bayes, and support vector machine. Among these classifiers, logistic regression (LR) showed effectiveness in identifying tweets. 2) Topic modeling using Latent Dirichlet Allocation (LDA) on nomophobia-relevant tweets classified by the LR model [10].
5.3.1.3 Findings:
The analysis, facilitated by the Latent Dirichlet Allocation (LDA) algorithm, revealed the existence of prominent themes in expressions of nomophobia. Before the pandemic, the primary themes included 'Addiction to Digital Entertainment' and frustrations due to 'Bad Internet Service.' However, during the COVID-era, the expressions transformed to include 'Work from Home Difficulties' and 'Extreme Attachment to Digital Technology' during quarantine [10].
5.3.1.4 Nomophobia Manifestation:
5.3.1.4.1 pre-COVID Era:
- Individuals demonstrated concern over unavailability and interruptions in digital entertainment services like Netflix and YouTube, symbolizing an inherent need to use the internet primarily for entertainment purposes [10].
- There was a significant expression of annoyance and impatience due to poor customer service related to internet and cellular services [10].
5.3.1.4.2 COVID-Era:
- The expressions morphed to depict the difficulties and concerns of people, especially IT professionals, teachers, and students, in working and studying from home due to poor internet connectivity [10].
- A novel dimension revealed was the extreme attachment to digital technology, where individuals felt lost, restless, and irritable when without a phone, emphasizing the heightened reliance on mobile technology during the pandemic and quarantine [10].
5.3.1.5 Implications:
This shift in nomophobia expression during the pandemic is reflective of the changing societal and professional norms, emphasizing the dependency on mobile technology not just for entertainment but also for professional obligations and social connectivity [10]. The loss of social connectivity has become a more pronounced factor in nomophobia, as reflected in the social media data analyzed in the study.
5.3.1.6 Conclusions:
The nomophobia study provides an insightful exploration of how nomophobia has manifested differently in response to the changing lifestyle and work paradigms induced by the COVID-19 pandemic. The use of social media data, specifically tweets, has proven to be effective in understanding the shifting paradigms in mobile phone dependency, showcasing the potential of such data in studying societal and behavioral changes [10].
5.3.1.7 Future Research:
Given the insights derived from this study, further exploration is warranted to examine whether the increased reliance on mobile technology for professional purposes and social connectivity persists post-pandemic and how it impacts societal relations and behaviors.
6. Conclusion and Future Directions:
In summary, the field of health care is experiencing growth, with the integration of machine learning (ML) and natural language processing (NLP). This progress is driven by advancements and ongoing research. NLP and ML could play a pivotal role in identifying mental health disorders like depression, anxiety, phobias, and more. There is potential for the development of real-time infrastructure, in this area. By analyzing language patterns, sentiment, and user interactions, these AI-driven tools can aid in early detection, providing individuals with timely support and guidance for better mental well-being.
References:
1) Zhang, T., Schoene, A.M., Ji, S. et al. Natural language processing applied to mental illness detection: a narrative review. npj Digit. Med. 5, 46 (2022). https://www.nature.com/articles/s41746-022-00589-7#Abs1
2) https://www.analyticsvidhya.com/blog/2022/07/the-evolution-of-nlp-from-1950-to-2022/
3) Khurana, D., Koli, A., Khatter, K. et al. Natural language processing: state of the art, current trends and challenges. Multimed Tools Appl 82, 3713-3744 (2023). https://doi.org/10.1007/s11042-022-13428-4
4) Gauld, Christophe, et al. "Comparison of relative areas of interest between major depression disorder and postpartum depression." Progress in Neuro-Psychopharmacology and Biological Psychiatry 121 (2023): 110671. https://www.sciencedirect.com/science/article/abs/pii/S0278584622001634?via%3Dihub
5) Le Glaz A, Haralambous Y, Kim-Dufor DH, Lenca P, Billot R, Ryan TC, Marsh J, DeVylder J, Walter M, Berrouiguet S, Lemey C. Machine Learning and Natural Language Processing in Mental Health: Systematic Review. J Med Internet Res. 2021 May 4;23(5):e15708. doi: 10.2196/15708. PMID: 33944788; PMCID: PMC8132982.
6) Rumshisky, A., Ghassemi, M., Naumann, T. et al. Predicting early psychiatric readmission with natural language processing of narrative discharge summaries. Transl Psychiatry 6, e921 (2016). https://doi.org/10.1038/tp.2015.182
7) S. Sulaiman, M. Mansor, R. Abdul Wahid, and N. A. A. Nor Azhar, "Anxiety Assistance Mobile Apps Chatbot Using Cognitive Behavioural Therapy", International Journal of Artificial Intelligence, vol. 9, no. 1, pp. 17-23, Jun. 2022
8) 1. Ahmed A, Hassan A, Aziz S, et al. Chatbot features for anxiety and depression: A scoping review. Health Informatics Journal. 2023;29(1). doi:10.1177/14604582221146719
9) Anmella G, Sanabra M, Primé-Tous M, Segú X, Cavero M, Morilla I, Grande I, Ruiz V, Mas A, Martín-Villalba I, Caballo A, Esteva J, Rodríguez-Rey A, Piazza F, Valdesoiro F, Rodriguez-Torrella C, Espinosa M, Virgili G, Sorroche C, Ruiz A, Solanes A, Radua J, Also M, Sant E, Murgui S, Sans-Corrales M, H Young A, Vicens V, Blanch J, Caballeria E, López-Pelayo H, López C, Olivé V, Pujol L, Quesada S, Solé B, Torrent C, Martínez-Aran A, Guarch J, Navinés R, Murru A, Fico G, de Prisco M, Oliva V, Amoretti S, Pio-Carrino C, Fernández-Canseco M, Villegas M, Vieta E, Hidalgo-Mazzei D Vickybot, a Chatbot for Anxiety-Depressive Symptoms and Work-Related Burnout in Primary Care and Health Care Professionals: Development, Feasibility, and Potential Effectiveness Studies J Med Internet Res 2023;25:e43293 URL: https://www.jmir.org/2023/1/e43293 DOI: 10.2196/43293
10) Visweswaraiah, Vaishnavi, et al. "Nomophobia before and after the COVID-19 Pandemic-Can Social Media be Used to Understand Mobile Phone Dependency." Int J Clin Med Info 4.1 (2021): 31-43. https://www.researchgate.net/publication/353851732_Nomophobia_before_and_after_the_COVID-19_Pandemic_-Can_Social_Media_be_Used_to_Understand_Mobile_Phone_Dependency
11) Padmavathy, R. D. "AI IN NOMOPHOBIA DETECTION: LEVERAGING ARTIFICIAL INTELLIGENCE TO IDENTIFY AND ADDRESS SMARTPHONE DEPENDENCY." EPRA International Journal of Multidisciplinary Research (IJMR) 9.7 (2023): 275-279.
12) Birnbaum, M. L., Norel, R., Meter, A. V., Ali, A. F., Arenare, E., Eyigoz, E., Agurto, C., Germano, N., Kane, J. M., & Cecchi, G. A. (2020). Identifying signals associated with psychiatric illness utilizing language and images posted to Facebook. NPJ Schizophrenia, 6. https://doi.org/10.1038/s41537-020-00125-0
13) Daraj, L.R.; AlGhareeb, M.; Almutawa, Y.M.; Trabelsi, K.; Jahrami, H. Systematic Review and Meta-Analysis of the Correlation Coefficients between Nomophobia and Anxiety, Smartphone Addiction, and Insomnia Symptoms. Healthcare 2023, 11, 2066. https://doi.org/10.3390/healthcare11142066
© 2025 ScienceTimes.com All rights reserved. Do not reproduce without permission. The window to the world of Science Times.