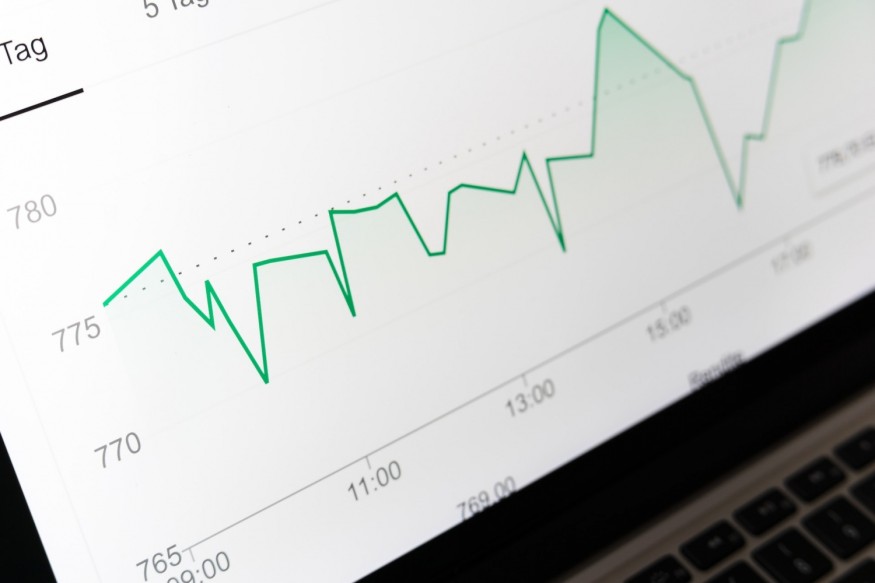
In the current business landscape, the integration of data analytics and machine learning (ML) algorithms has become a pivotal strategy for predicting future business performance and deriving actionable insights. Data analytics involves the systematic analysis of historical data using various tools and techniques to generate insights that aid in informed decision-making. When combined with ML algorithms trained on this historical data, these models can forecast future trends and patterns, revealing potential opportunities for businesses to enhance their performance.
Understanding Predictive Analytics
Predictive analytics, a key component of this approach, leverages current and historical data to anticipate future behaviors, outcomes, and trends. It employs a variety of statistical, modeling, data mining, and machine learning techniques to process and analyze data for prediction purposes. The primary goal is to go beyond knowing what has happened to provide the best assessment of what will happen in the future.
Machine Learning Algorithms in Predictive Analytics
- Regression Analysis: Often used in predictive analytics, regression models predict a continuous outcome. Linear and logistic regression are common types used for forecasting sales, revenue, and other business metrics.
- Decision Trees: These are used to create classification or regression models. They divide the dataset into subsets based on different criteria, each node of the tree representing a decision rule. This approach is particularly useful for complex decision-making processes in business.
- Random Forests: An extension of decision trees, random forests involve creating multiple decision trees and merging them together to get a more accurate and stable prediction. They are highly effective in handling large datasets with numerous variables.
- Neural Networks: Inspired by the human brain's structure and function, neural networks are particularly adept at identifying patterns and trends within large sets of data. They are increasingly used in complex scenarios like customer behavior prediction, risk assessment, and market trend analysis.
- Clustering Algorithms: These are used to group sets of data points into clusters based on similarity. This technique helps in market segmentation, identifying customer preferences, and targeting marketing strategies.
- Time Series Analysis: This involves analyzing time-ordered datasets to forecast future values based on previously observed values. Time series models are crucial in predicting stock market trends, economic forecasting, and inventory studies.
Benefits of Predictive Analytics in Business
- Improved Decision Making: Businesses can analyze historical data to make more informed decisions. Predictive analytics provide a foresight that can influence strategic planning and day-to-day decisions.
- Risk Reduction: Predictive models can identify potential risks and uncertainties in business operations. This enables businesses to develop strategies to mitigate these risks before they become significant issues.
- Enhanced Customer Experience: It's important to understand customer behavior patterns. This is how businesses can tailor their products, services, and interactions to meet the evolving needs and preferences of their customers, thus improving customer satisfaction and loyalty.
- Operational Efficiency: Predictive analytics can streamline business operations by forecasting demand, managing resources, and optimizing supply chains.
- Competitive Advantage: Businesses that effectively use predictive analytics can gain a competitive edge by identifying market trends, customer needs, and operational efficiencies before their competitors.
Challenges and Considerations
While the benefits are significant, businesses must navigate several challenges when implementing predictive analytics:
- Data Quality and Availability: The accuracy of predictions is highly dependent on the quality and completeness of the data.
- Model Complexity and Interpretability: Some ML models can be complex and difficult to interpret, which can be a barrier to understanding and trusting the predictions they make.
- Ethical and Privacy Concerns: The use of predictive analytics raises questions about privacy and the ethical use of data, especially in sensitive areas such as customer data.
- Constant Evolution: The business environment is dynamic, and models need to be regularly updated and retrained to remain relevant.
- Integration with Business Processes: Predictive analytics should be seamlessly integrated into the business processes for effective implementation.
The Future of Predictive Analytics
The future of predictive analytics in business looks promising, with advancements in ML algorithms and data processing technologies. The integration of artificial intelligence (AI) with predictive analytics is poised to enhance the accuracy and efficiency of predictions further. Additionally, as businesses continue to amass more data, the potential for deeper and more accurate insights grows.
Furthermore, the adoption of cloud computing and big data technologies is making predictive analytics more accessible to a broader range of businesses, including small and medium-sized enterprises. The increasing use of edge computing and IoT devices also presents new opportunities for real-time data analysis and forecasting.
Predictive analytics, powered by machine learning algorithms, offers businesses a powerful tool to forecast future trends, identify opportunities, and make informed strategic decisions. As technology evolves, its role in business strategy and operations is set to become even more significant, driving innovation, efficiency, and competitive advantage.

About Sudhahar Jayapalan
Sudhahar Jayapalan is a distinguished engineering leader in product development, boasting 23 years of experience at prominent companies such as Microsoft and Nokia. His career has been centered on data platforms, data analytics, artificial intelligence, cloud services, identity management, and mobile applications. At Nokia, Sudhahar played a pivotal role in developing consumer identity solutions to enhance personalized device experiences, while at Microsoft, he was instrumental in launching a sophisticated platform for data analysis and machine learning. His current focus is on designing complex systems for financial data management, harnessing the power of AI to offer adaptable and personalized insights into financial performance. A passionate advocate for diversity and inclusion, particularly promoting women in technology, Sudhahar is committed to mentoring and sharing knowledge, underscoring his belief in the transformative power of data, ML, and AI to improve productivity and solve complex challenges in the modern technological landscape.
Connect: https://www.linkedin.com/in/sudhaharjayapalan
The views and opinions expressed here are strictly personal and do not reflect the official stance or perspective of Microsoft.