Machine learning is a relatively recent field of study, yet it has brought progress to other areas. Currently, a team of researchers is looking to set standards for its use.
Recommendations for the new standards are outlined in the comment article "Reproducibility Standards for Machine Learning in the Life Sciences," appearing in the Nature Methods journal, August 30. In the paper, researchers from life sciences suggest making studies aided by machine learning to become more reproducible.
Researchers recommend making standards based on model and code publication, data, programming best practices, and workflow automation. With the available information, they believe that the research community could generate more reliable results on which other studies can build on and replicate once these standards are met.
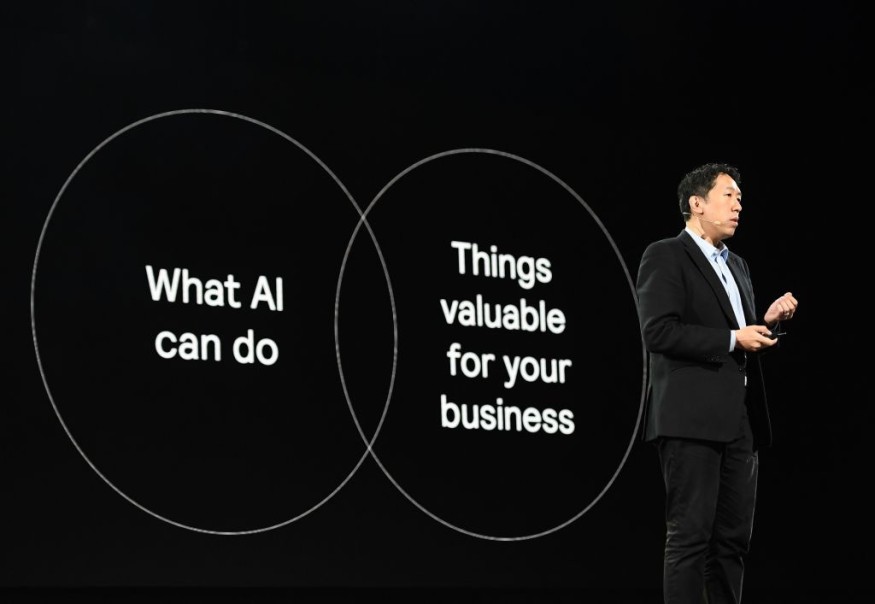
In the Name of Trust and Progress
The authors of the comment article also explain that setting standards are the key to advance scientific breakthroughs and continue to make advances, in addition to trust and reproducibility of a study.
One of the life sciences researchers in the new article is Casey S. Greene, Ph.D., the corresponding author and the director of the Center of Health at the University of Colorado School Medicine. He made the comment article with first author Benjamin J. Heil, a member of his research team, and other researchers in similar fields of study across the U.S., Canada, and Europe.
"Ultimately all science requires trust-no scientist can reproduce the results from every paper they read," authors of the paper noted. "The question, then, is how to ensure that machine-learning analyses in the life sciences can be trusted," they added, as reported by Tech Xplore.
A separate paper, appearing in the March issue of the Science Translational Magazine journal, titled "Reproducibility in Machine Learning for Health Research: Still a Ways To Go," evaluated more than 500 scientific papers aided by machine learning across different fields. The report noted that health-related works have the poorest reproducibility measures, based on data and code accessibility criteria.
To achieve this, researchers proposed a three-tiered system based on accessibility levels: Gold, Silver, and Bronze. Each class defines a set of minimum requirements for sharing study materials, allowing other scientists and researchers in the life sciences to trust and validate it if needed.
Gold, Silver, and Bronze Standards of Machine Learning
Researchers explain that the Bronze standard in life science studies would require publicly available data, code, and machine learning models. This level should allow other researchers to understand better and validate how a machine learning model performed its required tasks.
Usually, these models are first trained to recognize a specific trait or pattern by having them examine large datasets, according to Appen, a machine learning platform developer. The same datasets could also guide other researchers in training their respective machine learning models.
In the next level, Silver, the same standards from Bronze are required. However, it adds information about the system used for running the code. Theoretically, this should allow future researchers to duplicate the training process used in the reference study.
Lastly, Gold standards cover everything in the first two tiers plus an 'easy button' that should make it possible to reproduce the previous analysis and results with a simple command. All steps leading to the generation of the research via the machine learning model should be fully automated to minimize what authors called "the burden of reproducing their work."
RELATED ARTICLE : Machine Learning Might Guide the Arrow of Time in Microscopic Processes
Check out more news and information on Machine Learning in Science Times.