Implementing medical artificial intelligence (AI) into clinical and radiology practice has been limited mainly because of the cost, time, and expertise needed to accurately label imaging datasets. But there is a need to automatically and efficiently annotate large external datasets at a user-selected level of accuracy.
Researchers believe that retraining to improve the accuracy of existing AI models into an explainable, model-derived atlas-based methodology will help standardize the labeling of open-source datasets.
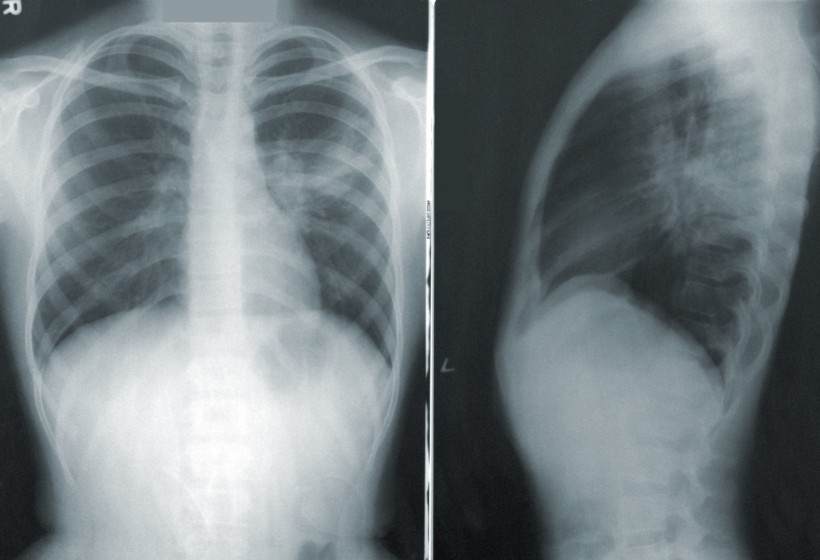
X-ray showing Pneumonia
What is an Explainable Artificial Intelligence (AI)?
As AI becomes more advanced each year, humans are finding it more challenging to comprehend and retrace its algorithm that the whole calculation process has become a black box that is impossible to interpret. Not even engineers or data scientists who create the algorithm can explain and understand what is happening inside and how the AI came to a result.
But explainable AI (XAI) can describe an AI model to help characterize model accuracy, fairness, transparency, and outcomes in AI-based decision-making, according to International Business Machines Corporation (IBM). XAI is a set of processes and methods that enable humans to comprehend and trust the results derived from machine learning algorithms.
It plays a crucial role in building trust and confidence when using AI in a certain field, such as in clinical or radiology settings. More so, it helps the organization adopt a responsible approach to AI development.
XAI led AI-enabled systems to specific output to help developers ensure that the system is working as expected and meets regulatory standards.
Most importantly, XAI is one of the key requirements in implementing responsible AI since its implementation in real organizations. It will help adopt AI responsibly and build systems based on trust and transparency.
Explainable AI Accurately Labels Chest X-Ray Images
In the study titled "Accurate Auto-Labeling of Chest X-Ray Images Based on Quantitative Similarity to an Explainable AI Model," published in Nature Communications, researchers used the XAI model to accurately label large datasets of publicly available chest X-ray images.
Health Imaging reported that the team used more than 400,000 chest X-ray images from over 115,000 patients. Researchers trained, tested, and validated the model and found that it could automatically label a subset of data with an accuracy equivalent to seven radiologists.
The team said that the results suggest a model that can achieve accuracy in line with human experts, making it a key factor in overcoming the limitations of AI implementation in clinical and radiology settings.
Corresponding author Dr. Synho Do from Massachusetts General Brigham points out that an accurate, efficient annotation of large medical imaging datasets is an important limitation training and implementation of AI in healthcare, so the use of XAI will greatly help in real-world clinical scenarios.
For the study, they tested the XAI model in labeling five chest pathologies, including cardiomegaly, pleural effusion, pulmonary edema, pneumonia, and atelectasis. The labels were compared to expert radiologists and found that the model achieved the highest probability-of-similarity (pSim) values, with accuracy at par with seven radiologists.
On the other hand, lower confidence in the label accuracy was noted for pulmonary edema and pneumonia, which are historically more subjective interpretations. Nonetheless, the fact that it produced pSim metrics performance based on a pre-selected level of accuracy enables researchers to fine-tune the model as required.
RELATED ARTICLE: Romantic Relationship With Robots: How Does AI Influence Human-Robot Interactions?
Check out more news and information on Artificial Intelligence in Science Times.