Researchers have developed an AI system called ProGen to create synthetic enzymes from scratch. During lab tests, some of these artificial enzymes performed as well as natural enzymes, even if their amino acid sequences were significantly different. This experiment shows that NLP technology, originally designed for text processing, can understand some biological principles.
The ProGen system, created by Salesforce Research, uses next-token prediction to form amino acid sequences into artificial proteins. Scientists believe this new technology could surpass direct evolution, a Nobel-winning protein design method, and revolutionize the field of protein engineering by accelerating the development of new proteins for various purposes, such as therapeutics and plastic degradation.
According to James Fraser, a professor of bioengineering and therapeutic sciences at the UCSF School of Pharmacy and co-author of a recent study published in Nature Biotechnology, the synthetic designs were found to be superior to those influenced by evolution. The paper had been accessible on BiorXiv since July 2021 and received numerous citations before being published in a peer-reviewed journal on Jan. 26th.
Revolutionary Enzymes
Fraser stated that the language model is acquiring knowledge of evolution, though not in the same manner as the conventional evolutionary process. The generation of specific traits can now be adjusted, allowing for the creation of enzymes with specific properties such as high thermal stability, tolerance to acidic conditions, or avoiding interaction with other proteins.
To develop the model, the researchers fed 280 million protein amino acid sequences into the machine learning system and allowed it to process the data for several weeks. Afterward, they fine-tuned the model by providing 56,000 sequences from five lysozyme families and additional context information regarding these proteins.
The model rapidly produced one million sequences, and the research team chose 100 to experiment on based on their similarity to natural protein sequences and the natural grammatical and semantic features of their underlying amino acids. From the initial 100 proteins, the team created five artificial proteins for testing in cells, which Tierra Biosciences screened. They compared the activity of these proteins to hen egg white lysozyme (HEWL), an enzyme present in chicken egg whites, and similar lysozymes found in human saliva, tears, and milk, which protect against bacteria and fungi.
Two of the five artificial enzymes could break down bacterial cell walls and had similar activity to HEWL, despite only having 18% identical sequences with each other and being around 70-90% identical to any known protein.
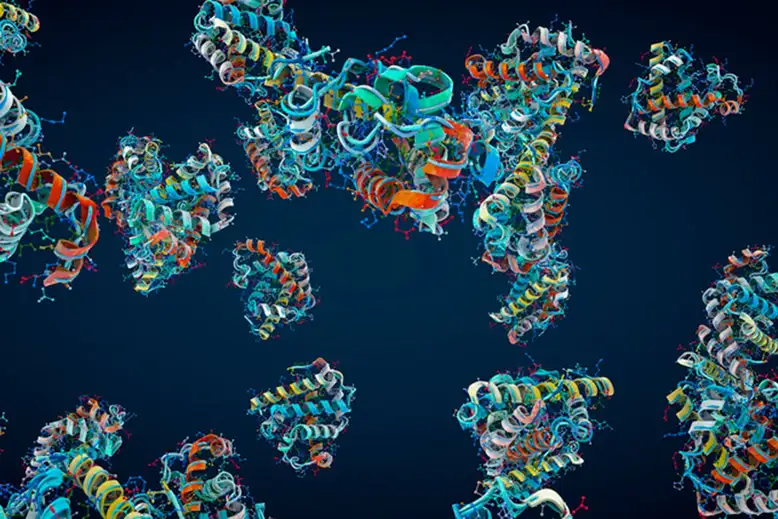
AI-Produced Enzyme Shape
Additionally, the researchers discovered that even when as little as 31.4% of their sequence was similar to any known natural protein, the AI-generated enzymes still showed activity, whereas just one mutation in a natural protein can render it inactive, as per Science Daily.
The AI system could deduce the appropriate enzyme shape by analyzing the raw sequence data. Using X-ray crystallography, the researchers found that the atomic structures of the artificial proteins were as they should be, even though the sequences were previously unseen.
ProGen was created by Salesforce Research in 2020 and is based on natural language programming initially developed for generating English text. The researchers, previously aware of the AI system's ability to learn grammar and the meaning of words and other writing composition rules, applied it to protein engineering.
According to Nikhil Naik, the Director of AI research at Salesforce Research and senior author of the paper, the sequence-based models have strong abilities to learn structure and rules when trained with lots of data, as they can understand what words can appear together and how they can be combined.
There are many possible combinations of amino acids in proteins, which makes the design choices for proteins almost limitless. Despite this, the AI model was able to generate working enzymes easily.
The capability to generate functional proteins from scratch is a significant milestone and marks the beginning of a new era in protein design, according to Ali Madani, the founder of Profluent Bio, a former research scientist at Salesforce Research, and the first author of the paper. This new tool is versatile and has the potential for therapeutic applications. The complete list of authors and funding information can be found in the paper, and a publicly available codebase for the methods described in the paper is also available.
RELATED ARTICLE: DeepMind Artificial Intelligence Database Predicts 3D Structures of Proteins Helping Scientists in the Field of Biology
Check out more news and information on Artificial Intelligence in Science Times.
© 2025 ScienceTimes.com All rights reserved. Do not reproduce without permission. The window to the world of Science Times.